By: Delthia Ricks
About five years ago, it became evident that certain high-tech soap dispensers in a growing number of restrooms failed to recognize dark skin. The electronic devices, which were being installed in airports, office buildings and other public venues, remained stubbornly unresponsive when Black people tried to wash their hands. But related problems soon grew increasingly more serious — and then potentially deadly. The artificial intelligence underlying facial recognition technology was found to more frequently misidentify people of color than whites. Worse still, the software embedded in some autonomous — self-driving — cars didn’t recognize dark skin tones, a flaw that could turn the futuristic vehicles into lethal weapons on the road.
As egregious as those examples are, they’ve emerged as only the tip of a massive iceberg. A quietly growing new form of racial discrimination is developing in the digital arena, prejudices that computer scientists define as algorithmic bias, bigotry embedded in the codes that drive the technology of daily life.
Algorithmic bias refers to unfairness or potential harm caused by skewed data in artificial intelligence systems. The growing list of discriminatory acts caused by algorithmic bias goes well beyond dispensing soap, self-driving cars or activating an iPhone.
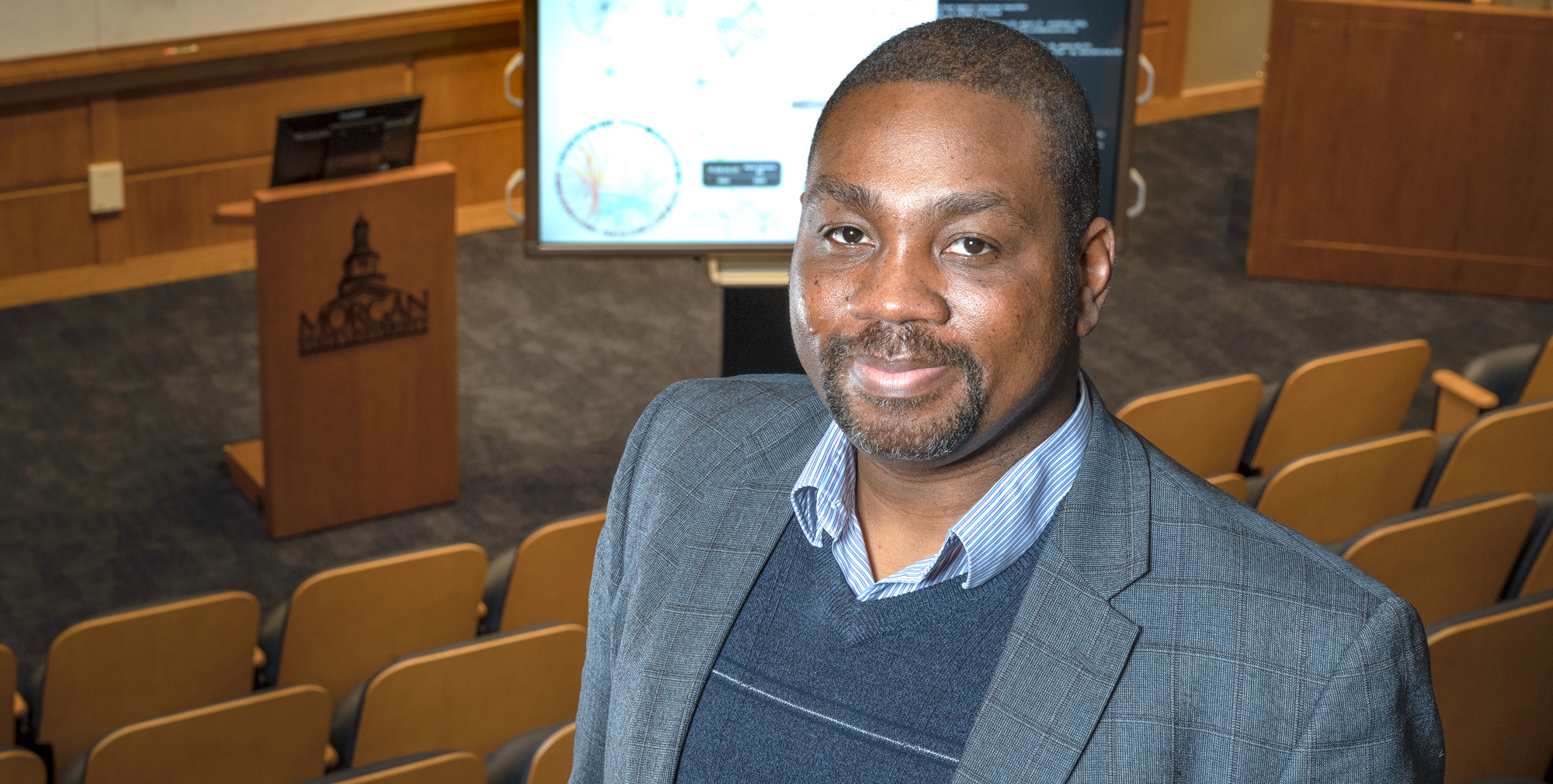
Our reliance on purely data-driven algorithms can exacerbate social inequality, and the question one must ask is, ‘Aren’t the people who design and deploy these systems even aware of its possible impact?’
— Kofi Nyarko, D.Eng
Leading AI Equity
“Those aren’t trivial concerns, but we are facing something much, much larger,” says Kofi Nyarko, D.Eng, professor in the Department of Electrical and Computer Engineering at Morgan State University. Dr. Nyarko is conducting research into algorithmic bias in collaboration with his Morgan colleague, Shuangbao (“Paul”) Wang, Ph.D., chair and professor of the Department of Computer Science. Following the recommendation of Morgan’s first-ever Blue-Ribbon Panel on STEM Research Expansion — a gathering of some of the country’s leading scholars and research scientists, held last December — the two have also founded a new national center at Morgan to address deep-seated problems in artificial intelligence (AI) systems that can lead to racist outcomes. “Most people would assume that we are talking about dispassionate systems, but that is not always the case. And that’s what can make AI so subversive,” Nyarko says.
Morgan’s new interdisciplinary Center for Equitable Artificial Intelligence and Machine Learning Systems (CEAMLS) specializes in mitigating algorithmic bias via “continuous research and engagement with academic and industrial leaders,” Nyarko adds. “One of the goals of the center will be to conduct research on how to develop socially responsible artificial intelligence and how to implement socially responsible AI systems.”
The new center is partnering with the Urban Health Disparities Research and Innovation Center at Morgan. “We will help them address health disparities and learn how algorithmic bias can creep into health (delivery and insurance) systems,” says Nyarko, noting that healthcare is an area where discriminatory data in an AI system has the potential to be life-threatening.
Funding for CEAMLS has already begun to roll in, starting with a $3-million grant from the State of Maryland. “We will receive this appropriation every year in perpetuity. But we are also seeking private funding,” Nyarko says.
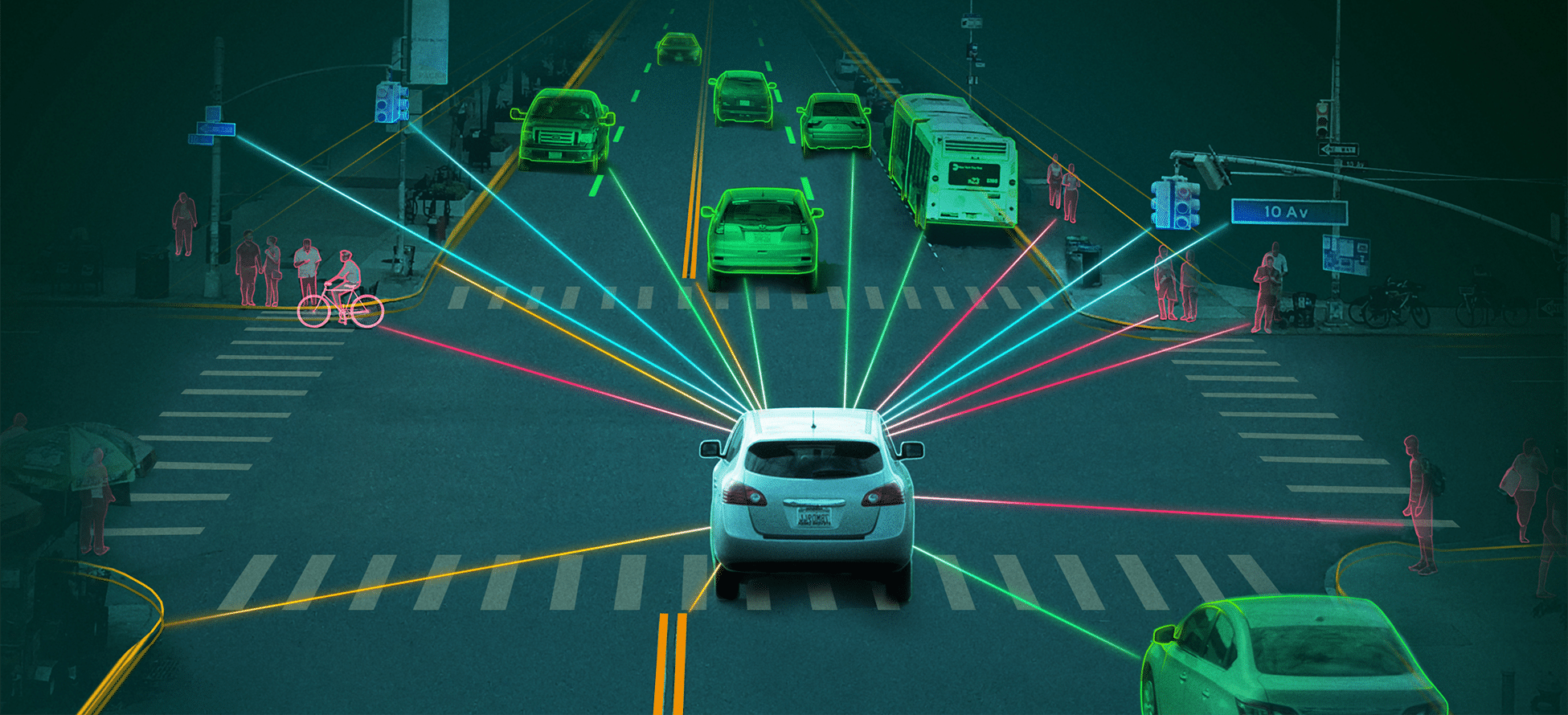
Broad Racial Impact
Yet he and Dr. Wang acknowledge facing uncharted terrain when it comes to addressing algorithmic bias, because, as both professors underscore, the tentacles of the problem run long and deep throughout myriad data systems involving diverse aspects of life.
“Our reliance on purely data-driven algorithms can exacerbate social inequality, and the question one must ask is, ‘Aren’t the people who design and deploy these systems even aware of their possible impact?’ ” Nyarko says.
By design, there are supposed to be checks and balances within artificial intelligence systems to weed out problematic data.
“Machine learning can reveal patterns that are difficult for human beings to uncover, which is done by analyzing data efficiently and accumulating knowledge gained from previous learnings,” Nyarko adds. However, the accuracy of algorithms can be affected by many factors, and one of the most destructive is “unintentional and intentional prejudices or biases,” he explains.
Problems involving autonomous cars were fortunately discovered years before the vehicles rolled onto streets. A 2019 study by scientists at Georgia Institute of Technology revealed the AI systems that powered the navigation of multiple models were less likely to recognize people with darker skin. None of the cars were in use, but the team of scientists found eight different models that had the same potentially deadly flaw: they kept moving against targets in the study that corresponded with a range of darker skin tones. If released on the road, the cars could have mowed down Black and Brown pedestrians.
The soap dispensers had a similar but far less dangerous problem. Because of a mere engineering issue, Nyarko says, the dispensers couldn’t recognize dark-colored skin. Light skin tones reflected light back to the device, activating the release of soap. Because dark skin absorbs more light, the device couldn’t be switched on, and Black people’s attempts at hand hygiene were impaired.
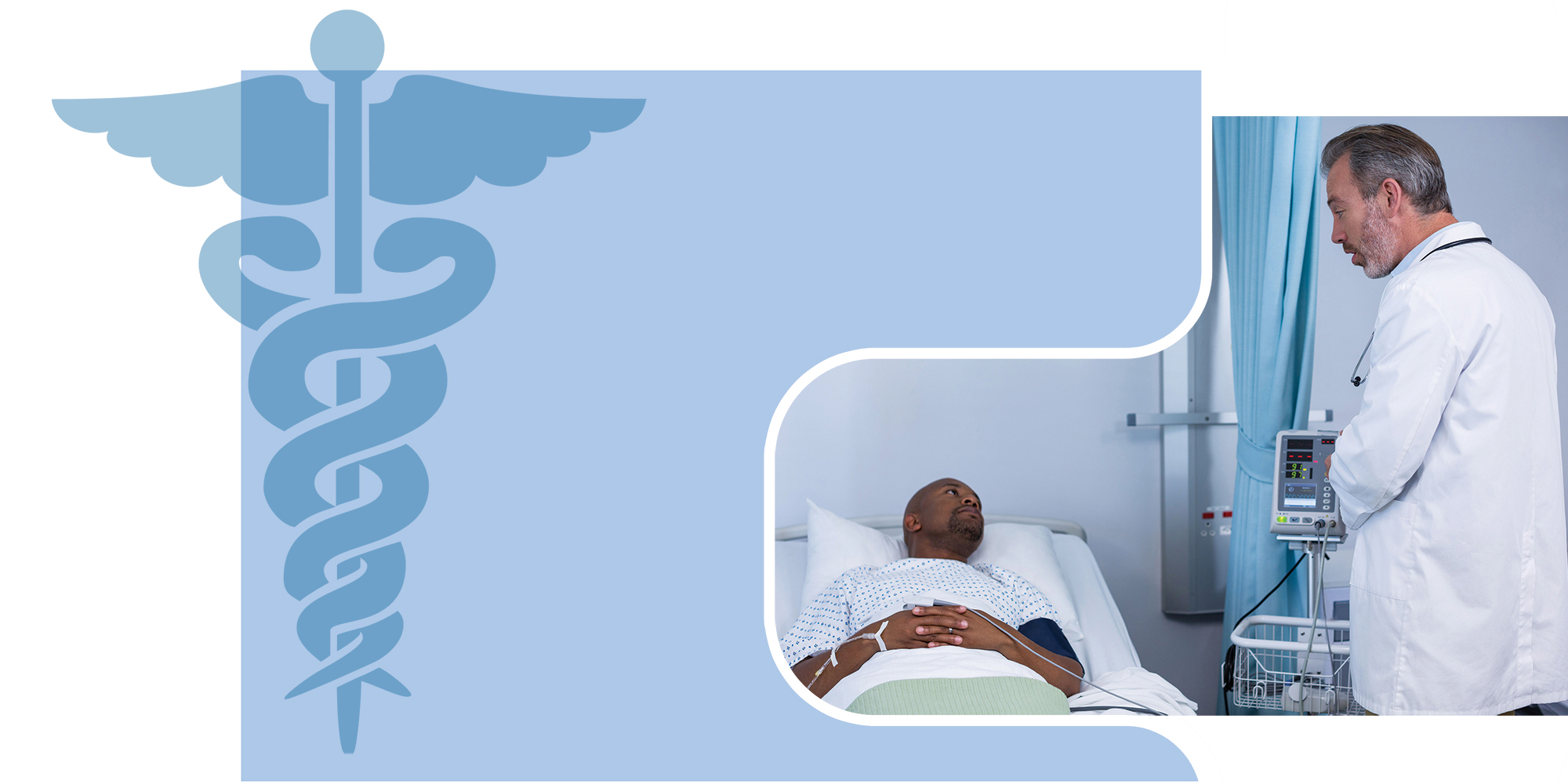
Healthcare Risks
Nowhere is algorithmic bias more glaring than in AI systems that determine the kind of healthcare patients receive. In his assessment of a 2019 study that unearthed racism in medical care, Nyarko asserted that inherently bigoted data hampered treatment for Black patients.
“A widely used commercial healthcare algorithm reflected a serious racial bias,” Nyarko explains, because it relied on the amount of money spent on individuals’ healthcare, with money serving as a proxy for health status. If less money was being spent on patients, then the system assumed those patients were healthier and had no need for routine or follow-up care.
Although the system was designed to curb costs for the healthcare provider, a deep dive into its data uncovered a disparity that has long characterized racial differences in U.S. healthcare: on average, less money is spent on Black patients. Even though Black patients’ need for medical care may have been the same as or greater than that of their white counterparts, the algorithm falsely concluded that Black patients were healthier, because less money was being spent on the battery of tests, hospitalizations, medications and other treatments that whites received routinely.
“Black patients were consistently and significantly more sick than white patients,” adds Nyarko, but the biased algorithm influenced who was deemed more worthy of care on the basis of spending. For Black patients, that meant vital treatment denied.
“The developers of these algorithms and the AI models that drive them should be made aware of the impact of the choices they make along the entire life cycle of their systems,” Nyarko asserts. “There needs to be a sustained focus on the prevention of algorithmic bias.”
Sustained Prevention
Other examples of algorithmic bias abound.
Research in recent years has found that online mortgage companies can be as biased as face-to-face lenders. A 2018 analysis by researchers at the University of California, Berkeley found inherent biases in the artificial intelligence systems of online lenders, which resulted in Black and Latino homebuyers’ being charged higher fees.
Prejudicial lending practices of the past mainly grew from in-person meetings of homebuyers and lenders at a bank. But disparities now are more frequently the result of formulas in machine learning — algorithms — that incorporate many of the same prejudices. The UC Berkeley research found that Black and Latino borrowers paid up to a half-billion dollars more in annual interest compared with white borrowers who had comparable credit scores.
Yet the problems with algorithmic bias don’t end there. Troubled facial recognition technology resulted in flawed iPhones in 2017 that didn’t discern the faces of Asian women, enabling almost any Asian woman to unlock another’s phone. More complicated still, research by the U.S. Department of Commerce has found that facial recognition technology is more likely to misidentify people of color than whites. If used by law enforcement, the data-driven system could result in wrongful arrests, the study found.
“The developers of these algorithms and the AI models that drive them should be made aware of the impact of the choices they make along the entire life cycle of their systems,” Nyarko asserts. “However, since the field of data science and AI is continuously evolving, it is not a process that can be examined just once and then put into practice. There needs to be a sustained focus on the prevention of algorithmic bias.”